Insights
Get insights into the latest happenings at Manhattan or filter content to learn more about a specific topic.
Featured
Gartner Magic Quadrant for WMS
Manhattan is a 17-time Leader in Gartner’s Magic Quadrant for WMS, recognized for its cloud-native, microservices-based warehouse management solutions.
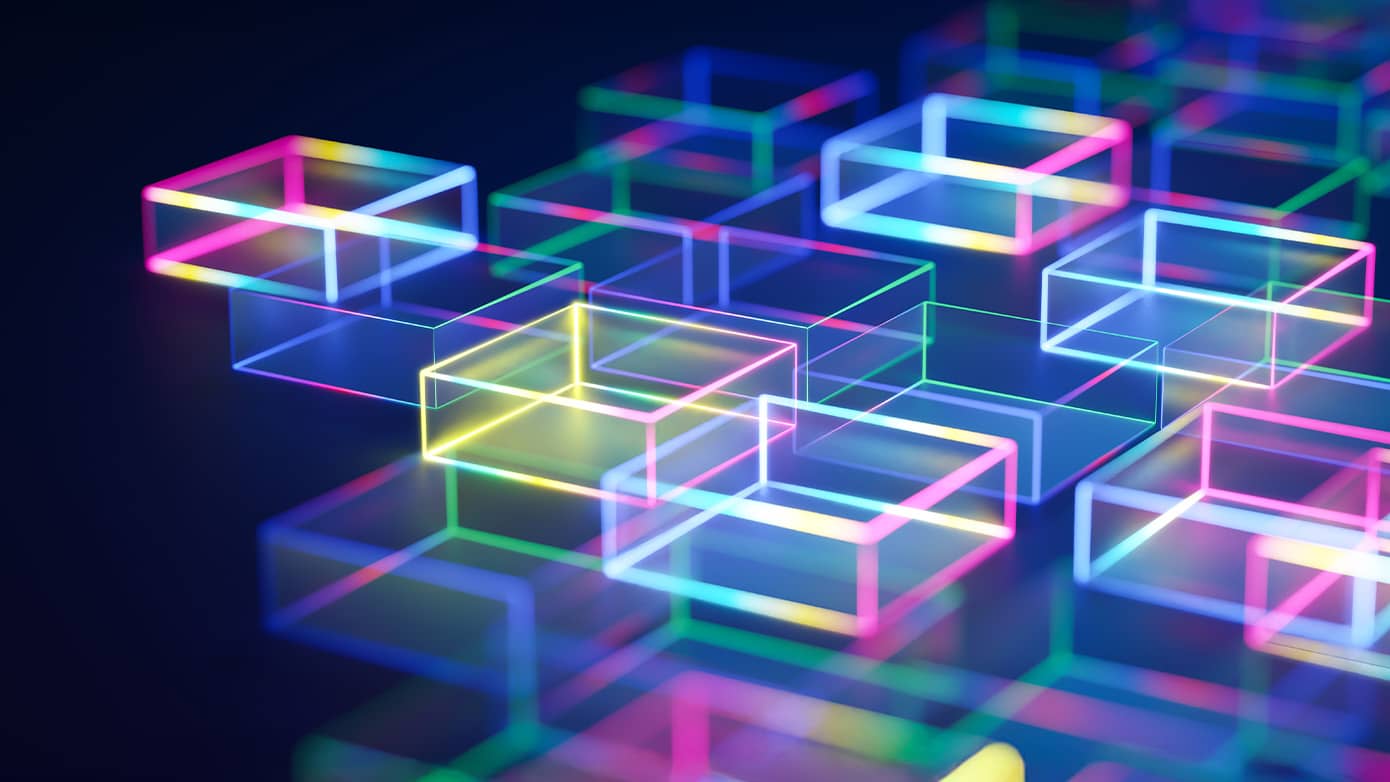