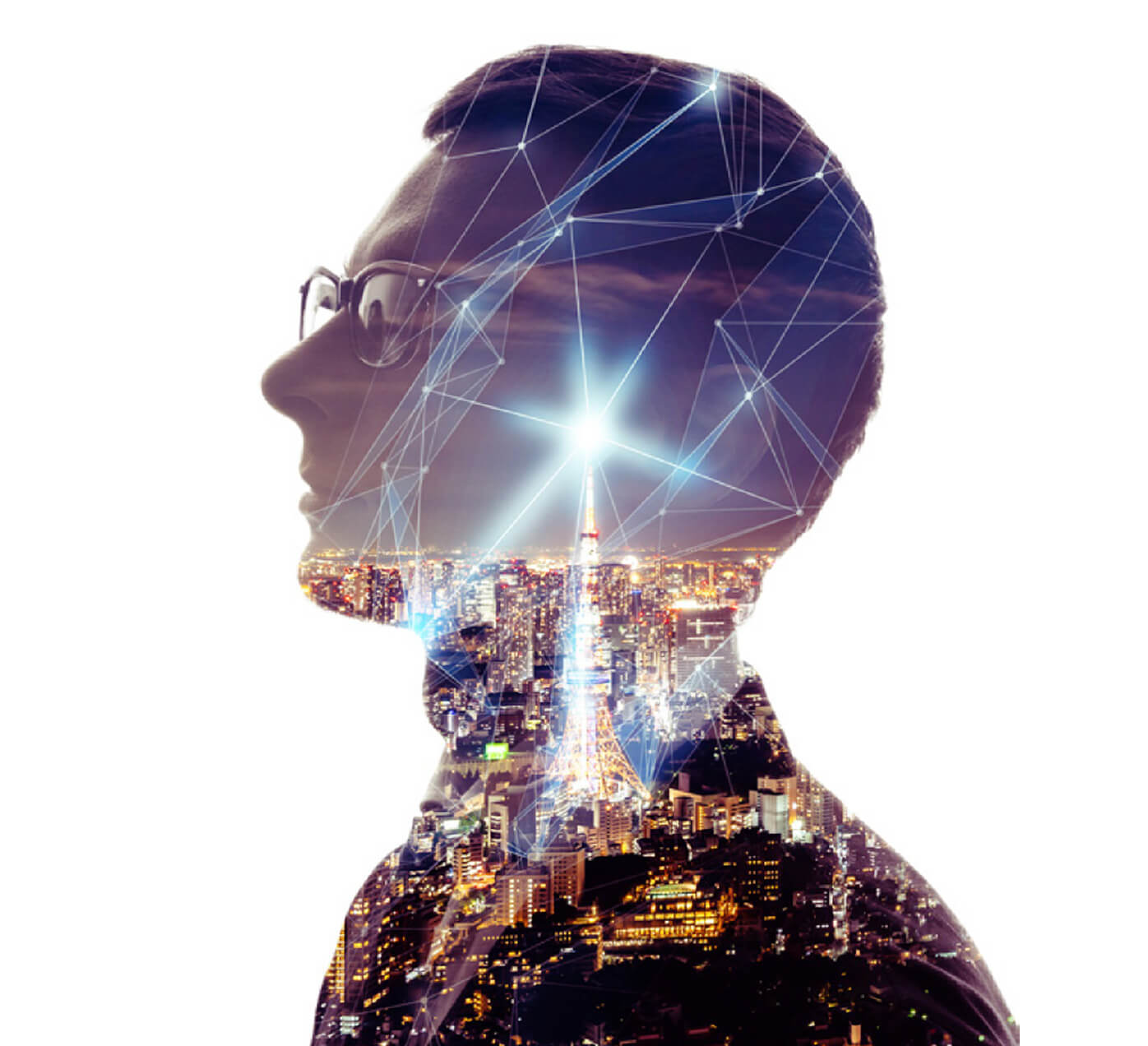
The Evolution of Demand Forecasting
Forecasting demand has always been a critical yet challenging aspect of supply chain management, and over the past decade, this challenge has intensified.
- Rapid shifts in consumer preferences driven by shorter product life cycles and social influences.
- The rise of omnichannel commerce, requiring seamless integration across in-store, online and delivery operations.
- Increasing unpredictability from external factors, such as geopolitical events, economic fluctuations, and climate change.
The limitations of traditional methods have resulted in significant inefficiencies.
- Overstocks, wasted resources, and markdowns.
- Stockouts, lost sales, and dissatisfied customers.
- Ineffective promotions that don’t align with real-time demand.
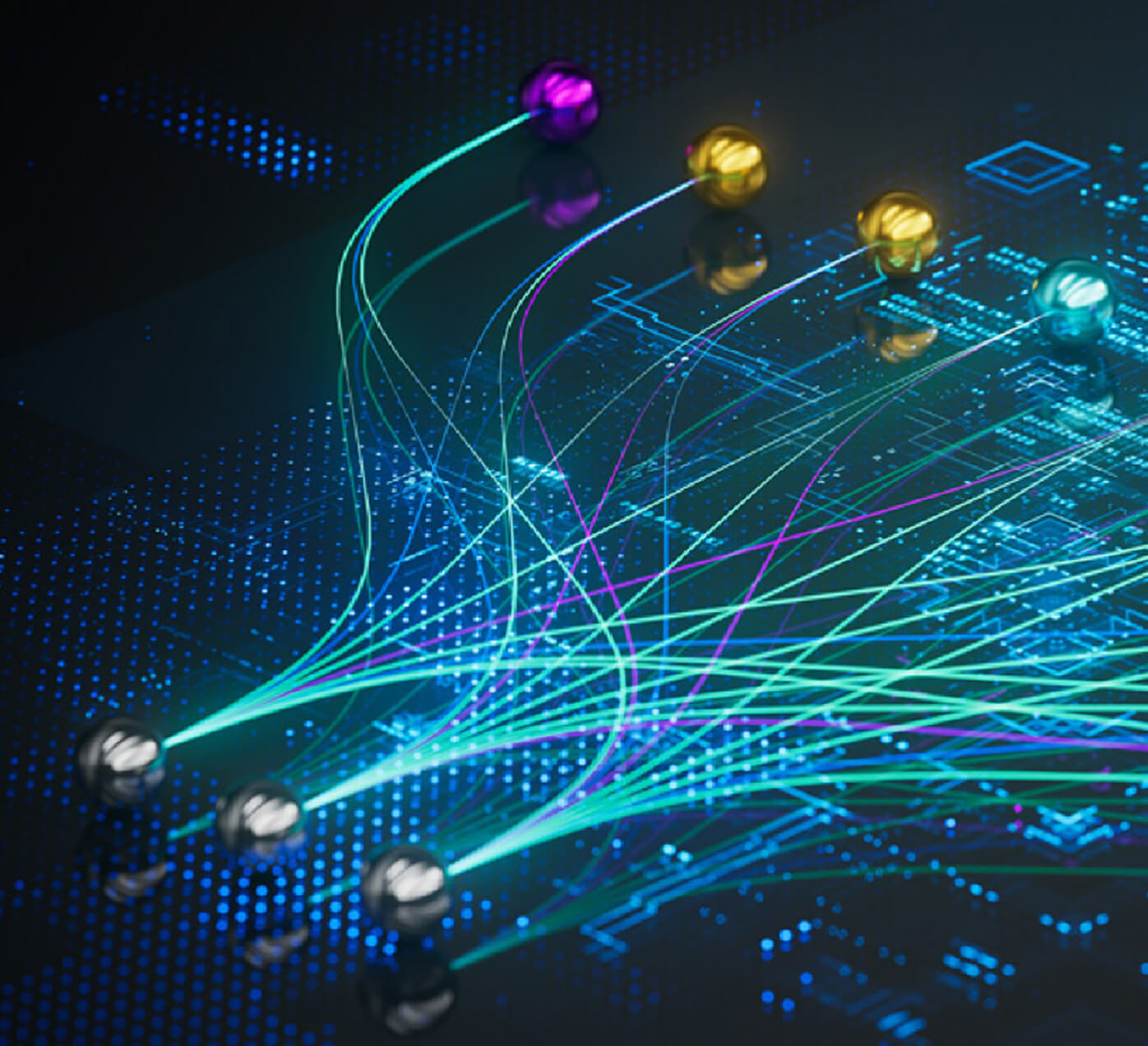
Reliable, But Can it be Ready for the Unexpected?
Strengths
- Proven accuracy for certain demand patterns
Ideal for seasonality, intermittent demand, and short-term fluctuations. - Transparency & interpretability
Easily explainable models that enable better decision-making and regulatory compliance. - Computational efficiency & faster execution
Lower cost, faster processing, and easier deployment compared to AI-driven methods.
Weaknesses
- Lack of adaptability
These models struggle to account for rapid changes in demand caused by promotions, market trends or external disruptions. - Data dependency
Reliance on historical data limits their ability to incorporate real-time, external factors. - High complexity
Tailoring multiple models for diverse products and demand patterns becomes cumbersome as portfolios grow.
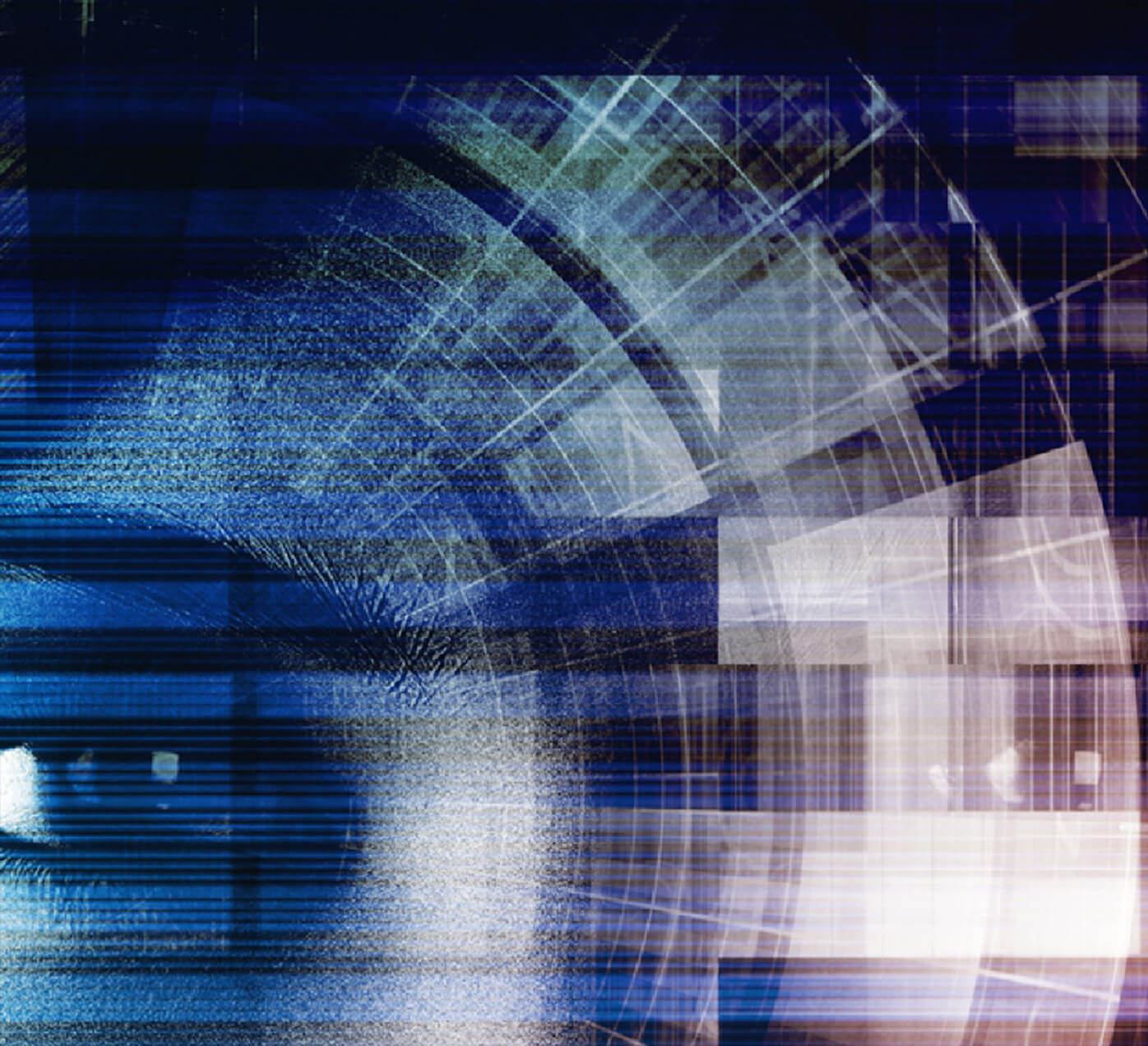
A Game-Changer, But Not the Whole Game
Strengths
- Nonlinear pattern detection AI excels at identifying
- intricate relationships between variables, such as the impact of weather on sales.
- Integration of external data AI can incorporate diverse sources like social media trends, economic indicators, and competitor actions.
- Dynamic learning Continuous adaptation to new data ensures forecasts remain relevant.
Weaknesses
- Data quality dependency Poor-quality or sparse data can compromise accuracy.
- Resource-intensive Implementation requires significant computational power and expertise.
- Overfitting risks AI models may focus excessively on irrelevant patterns, leading to inaccurate predictions.